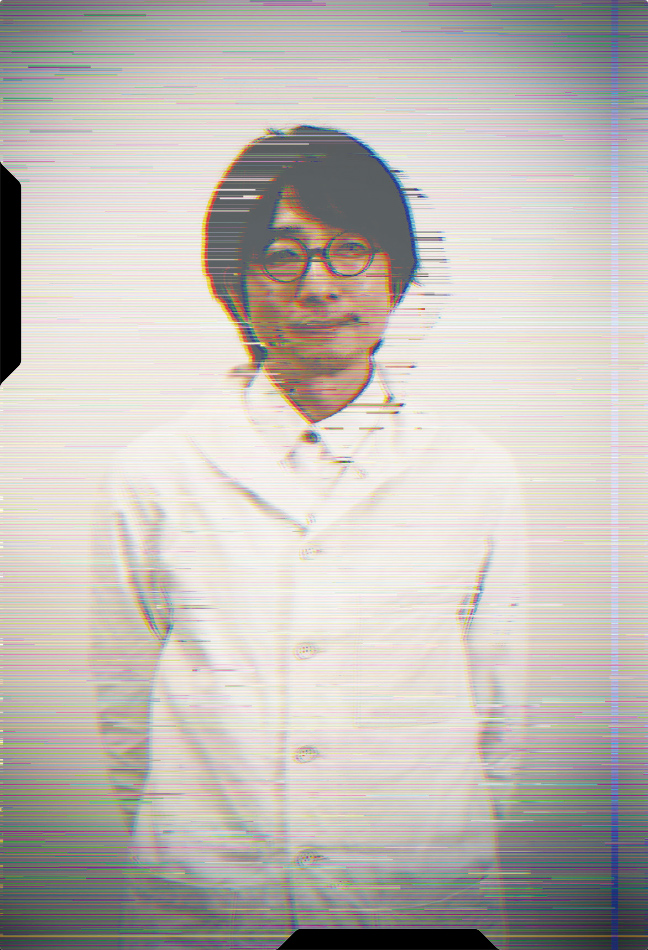
Many people think that science will eventually be able to explain everything that happens in nature, and that technology will be able to reproduce it. Perhaps that is so, but even then, that day lies far into the future. Probably a more likely scenario is that the further science and technology advance, the deeper the mysteries of the world will grow. Even with topics that we believe science has solved for good, when you take a closer look, you'll find that plenty of problems have slipped through the cracks or been swept under the carpet. Furthermore, these are often the issues that are closest to us and most important in our daily lives. Take hunches or intuitions or premonitions, for example. They may have rational-sounding explanations, but our gut feelings tell us something is not quite right after all. Such examples are not at all uncommon. When you think about it, there are lots of things that modern civilization has forgotten all about. Maybe the time has come to stop for a moment and try to remember. The seeds of forthcoming science and technology are impatiently waiting to be discovered among the things we have left behind.
HORIBA, Ltd.
Ever since fully-fledged research in artificial intelligence began in the middle of the 20th century, some of the wisest, most naturally intelligent scientists of each age have been involved in its development. They have also had to confront tricky issues like what it means to be human, and what intelligence really is – major questions that humankind has been asking almost since the dawn of civilization.
Natural language processing and image recognition are seen as essential technologies to bring AI closer to humans. However AI (technology) can’t process the meaning and nuances of words or meanings. It can only process words and images that have been transformed to strings of ones and zeros. Even if you store a vast number of example sentences, or increase the image resolution, the system still can’t make a “natural” judgment. Perhaps it’s better to regard these technologies as ways of getting humans used to artificial judgments.
Alan Turing, who is considered the father of computer science, proposed a test in 1950 to determine whether a machine was intelligent or not, the so-called Turing Test: A human judge uses natural language to conduct a dialogue without direct interaction with the other party, and then determines whether the other party is intelligent or not. However, even if the other party is another human being and communication is established, it is sometimes only the other person himself/herself who knows whether he or she really is intelligent. For that matter, we often sense signs of intelligence in cats and dogs, who don’t use human language.
>>> Neurology: “The brain is an electrical network of nerve cells”
>>> Norbert Wiener: Cybernetics
>>> Claude Shannon: Information theory
>>> Alan Turing: Theory of computation → The digitalization of computation
>>> Walter Pitts & Warren McCullough: Analysis of networks of idealized artificial neurons → Neural networks
The term “artificial intelligence” was coined by the cognitive scientist John McCarthy at the Dartmouth conference in 1956. Originally, the term referred to a man-made object with an intelligence equal or superior to that of a human being, but today the meaning has been extended to comprise various technologies developed toward that general goal. That is to say, AI = Artificial Intelligence, but more often AI = Artificial Intelligence technology. The definition of “intelligence” is vague, though, and we still don’t have any method to scientifically measure human intelligence, so actual “artificial intelligence” is not about to be realized any time soon.
>>> 1950 Alan Turing: The Turing Test
>>> 1951 Marvin Minsky: The neural network machine SNARC
>>> 1951 Christopher Strachey: Checkers program / Dietrich Printz: Chess program → Game AI
>>> 1955 Allen Newell & Herbert Simon: “Logic Theorist” → Strong AI
>>> 1956 Dartmouth Conference: Proposal of the term “Artificial Intelligence”
“Weak AI” refers to systems that can solve problems and make inferences, without requiring the full cognitive abilities of a human. “Strong AI” refers to systems that approach human intelligence or can perform human tasks, and furthermore possess a broad knowledge and some sort of self-consciousness.
Herbert Simon & Allen Newell:
“Within a decade, a computer will beat the world champion at chess, and discover and prove important mathematical theorems.” (1958) Herbert Simon:
“Within 20 years, machines will be able to do everything that humans can do.” (1965) Marvin Minsky:
“Most problems in order to generate artificial intelligence will be solved within the next generation.” (1967) Marvin Minsky:
“Machines with the general intelligence of average human beings will emerge within the next three to eight years.” (1970)
>>> 1957 Frank Rosenblatt: Perceptron → Connectionism (Realization and implementation of intelligent bodies based on a neural network model)
>>> The STUDENT program
>>> First AI programs using semantic networks
>>> Joseph Weizenbaum: ELIZA (Natural language approaches)→ Chatbots
>>> Marvin Minsky & Seymour Papert: Micro-World proposal
Expert systems became the core technology of the second AI boom in the 1980s. The idea was to make a computer gain the knowledge of a specialist such as a lawyer or a doctor, and eventually replace the specialist. However, in actual medical practice, for example, patients often complain of general malaise and other problems that are difficult to define, and various unspecified peripheral information such as knowledge of the patient’s preferences or family circumstances may also affect the doctor’s diagnosis and way of solving the problem to some extent. In the end, the limits of expert systems were revealed, and the second AI boom faded out again.
>>> Limitations of computer power: Insufficient memory and processing speed
>>> Combinatorial explosion due to intractability
>>> Limitations of common sense knowledge and reasoning
>>> Moravec’s paradox: “Sensorimotor skills require more computational resources than advanced reasoning.”
>>> The Frame Problem and the Conditional Assignment Problem
One of the most important challenges in AI development is the so-called Frame Problem. A computer with limited information processing capacity cannot deal with all problems that might actually occur. Taking everything into consideration requires infinite time, so the processing has to be limited to what can be done within a given frame. However, since both science and mathematics rely on setting boundary conditions, this problem may be impossible to solve. Exactly how humans resolve this issue has not yet been elucidated.
>>> Limitations of expert systems
>>> End of 1980s: Robotics-based approaches → Revival of cybernetics and control theory
>>> 1980 The expert system XCON
>>> 1981 The Japanese “Fifth Generation Computer Project”
>>> 1982 John Hopfield: Backpropagation → Revival of connectionism
>>> 1984 The Cyc project
……An attempt to create a database of common sense knowledge and construct a system capable of human-like reasoning
A name that brings back memories to people of a certain age, the Fifth Generation Computer project was a national project launched by the Japanese Ministry of International Trade and Industry in 1982, proudly proclaiming its aim to create an artificial intelligence surpassing human intelligence. It made quite a stir worldwide, but after 10 years and a budget of 57 billion yen, the hoped-for natural language processing capabilities had not materialized, and the concrete results were few. The only thing that was completed was a parallel processing system with hardly any applications to run on it.
Big Data refers to vast data sets that exceed the capabilities of ordinary software. Instead, statistics, pattern recognition, and AI analysis methods are utilized to discover and extract information from these huge amounts of data, technologies known as “data mining.” Together with “cloud computing,” Big Data has been one of the major buzzwords of the 2010s.
The application of Big Data to AI development is often pointed out, and be that as it may. Humans, on the other hand, are able to draw conclusions based on very small amounts of data.
>>> 1990s “Intelligent agents” that act independently, learn, and adapt to the circumstances
>>> 1997 Deep Blue defeats the World Champion of Chess.
>>> 2005 A robot car wins the DARPA Grand Challenge.
>>> 2005 Ray Kurzweil’s The Singularity is Near
The futurist Ray Kurzweil claims that the “technological singularity” will arrive around the year 2045. It is the point when an AI will be able to create an even more powerful AI by itself, without human assistance. From that moment on, AIs will be the protagonists of civilization. The Japanese AI researcher Motoaki Saito has suggested that we will reach a “pre-singularity” (social singularity) already in 2025, when food, clothing and shelter will be available for free, and immortality will also be made possible. Naturally, many skeptic voices have been raised toward both the pre-singularity and the singularity, and their timing.
>>> 2006 Geoffrey Hinton: Deep learning
>>> 2010 Big Data
>>> 2011 IBM’s Watson system participates in the quiz game show Jeopardy and defeats two champions.
>>> 2013 A robot takes the entrance exam to the University of Tokyo.
>>> 2014 The weak AI chatbot Eugene Goostman passes the Turing Test.
>>> 2016 AlphaGo defeats a human pro Go player.
Deep Learning is a branch of machine learning and a technology that uses multi-layer neural networks modeled on brain circuits, allowing the computer to capture characteristic features in the data and make more accurate and efficient judgments. Since the early 2010s, programs based on Deep Learning have advanced rapidly, and caught the public eye when a program using this technology defeated one of the world’s top professional Go players. After the appearance of Deep Learning, discussions about the singularity have started taking on a certain degree of reality.